What is Natural Language Processing (NLP)?
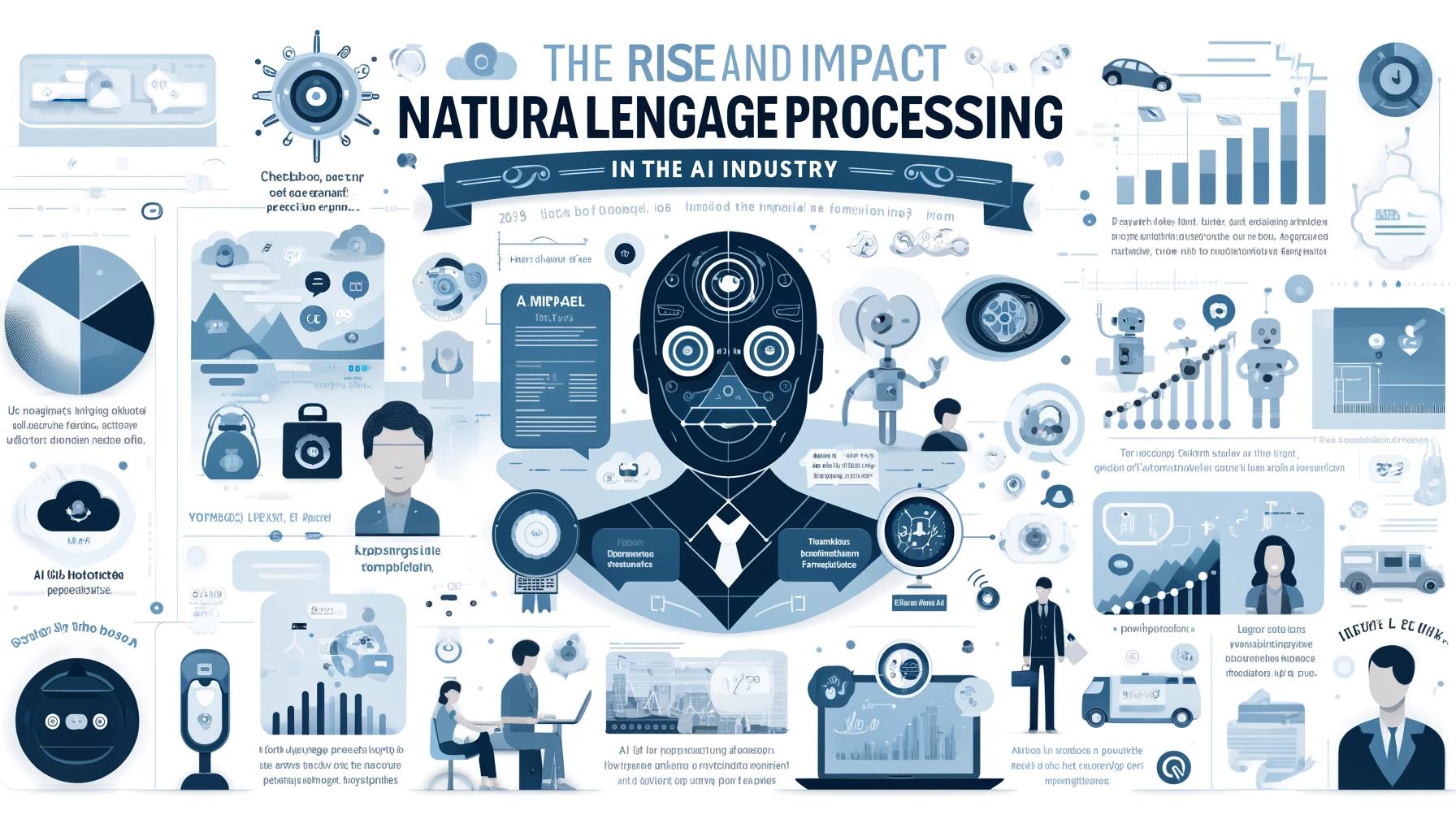
Natural Language Processing (NLP) is one of the most transformative technologies in the artificial intelligence (AI) industry. This branch of AI focuses on the interaction between computers and humans through natural language. Over recent years, NLP has made significant strides, becoming an integral part of various applications that enhance our daily lives. From virtual assistants and chatbots to advanced text analysis and sentiment detection, NLP is reshaping how we interact with technology.
The Evolution of Natural Language Processing
NLP has come a long way since its inception. Initially, it was limited to basic text recognition and simple keyword matching. However, with advancements in machine learning and deep learning, NLP has evolved to understand and generate human language more accurately and contextually. Key milestones in NLP development include:
-
Rule-Based Systems: Early NLP systems relied on handcrafted rules and heuristics to process language.
-
Statistical Models: The introduction of statistical methods allowed NLP systems to learn from large datasets, improving accuracy and flexibility.
-
Deep Learning: The advent of neural networks and deep learning algorithms, particularly transformers, has revolutionized NLP. Models like BERT, GPT-3, and GPT-4 have demonstrated remarkable capabilities in understanding and generating human language.
Applications of NLP
NLP's applications are vast and varied, impacting numerous industries and enhancing various technological solutions:
-
Chatbots and Virtual Assistants: NLP powers intelligent chatbots and virtual assistants like Siri, Alexa, and Google Assistant, enabling them to understand and respond to user queries in a natural, conversational manner. These technologies are widely used in customer service, home automation, and personal productivity.
-
Text Analysis and Sentiment Analysis: Businesses use NLP to analyze vast amounts of text data from social media, customer reviews, and feedback. Sentiment analysis helps companies gauge public opinion, improve products, and tailor marketing strategies.
-
Language Translation: Tools like Google Translate leverage NLP to provide real-time translation services, breaking down language barriers and facilitating global communication.
-
Healthcare: NLP applications in healthcare include the analysis of medical records, research papers, and clinical trial data to extract valuable insights. It also aids in developing conversational agents that assist patients with their healthcare queries.
-
Finance: NLP is used in the finance sector for analyzing market trends, automating trading strategies, and detecting fraudulent activities by examining textual data from various sources.
NLP Technologies and Innovations
Several innovative NLP technologies have emerged, driving the field forward:
-
Transformer Models: Models like BERT (Bidirectional Encoder Representations from Transformers) and GPT-3 (Generative Pre-trained Transformer 3) have set new benchmarks in NLP performance. These models use attention mechanisms to understand the context and relationships between words in a text, allowing for more accurate language understanding and generation.
-
Generative AI: Advances in generative AI have led to the creation of models capable of producing human-like text. For example, GPT-4 can generate coherent and contextually relevant text, making it useful for content creation, code generation, and even creative writing.
-
Multimodal NLP: This emerging field combines text, audio, and visual data to create more comprehensive and context-aware AI systems. Applications include video analysis, interactive educational tools, and enhanced virtual assistants that can understand and respond to a variety of inputs.
Challenges and Future Directions
Despite its advancements, NLP still faces several challenges:
-
Ambiguity and Context: Human language is inherently ambiguous and context-dependent, posing significant challenges for NLP systems. Understanding nuances like sarcasm, idioms, and context-specific meanings requires sophisticated models and large datasets.
-
Bias and Fairness: NLP models can inadvertently learn and propagate biases present in training data. Ensuring fairness and mitigating bias in NLP systems is crucial for their ethical deployment.
-
Multilingual Capabilities: While significant progress has been made in NLP for major languages, achieving high accuracy across all languages and dialects remains a challenge.
Looking forward, the future of NLP will likely focus on:
-
Improving Contextual Understanding: Continued development of models that can better understand and generate contextually accurate language.
-
Enhancing Multilingual NLP: Expanding NLP capabilities to support more languages and dialects, enabling truly global applications.
-
Ethical AI: Developing frameworks and guidelines to ensure the ethical use of NLP technologies, addressing issues like bias, privacy, and transparency.
Natural Language Processing is a cornerstone of modern AI, driving innovations that enhance how we interact with technology. From virtual assistants and chatbots to advanced text and sentiment analysis, NLP's applications are vast and impactful. As technology continues to evolve, addressing its challenges and harnessing its potential will be key to shaping the future of AI and its integration into various aspects of our lives.
References
1. Crunchbase: AI Startups Hot in 2023
2. Techopedia: AI Startup Investments Surge in 2023
3. Visual Capitalist: Ranked AI Startups by Country