Decoding the t-Test: A Guide to Comparing Means
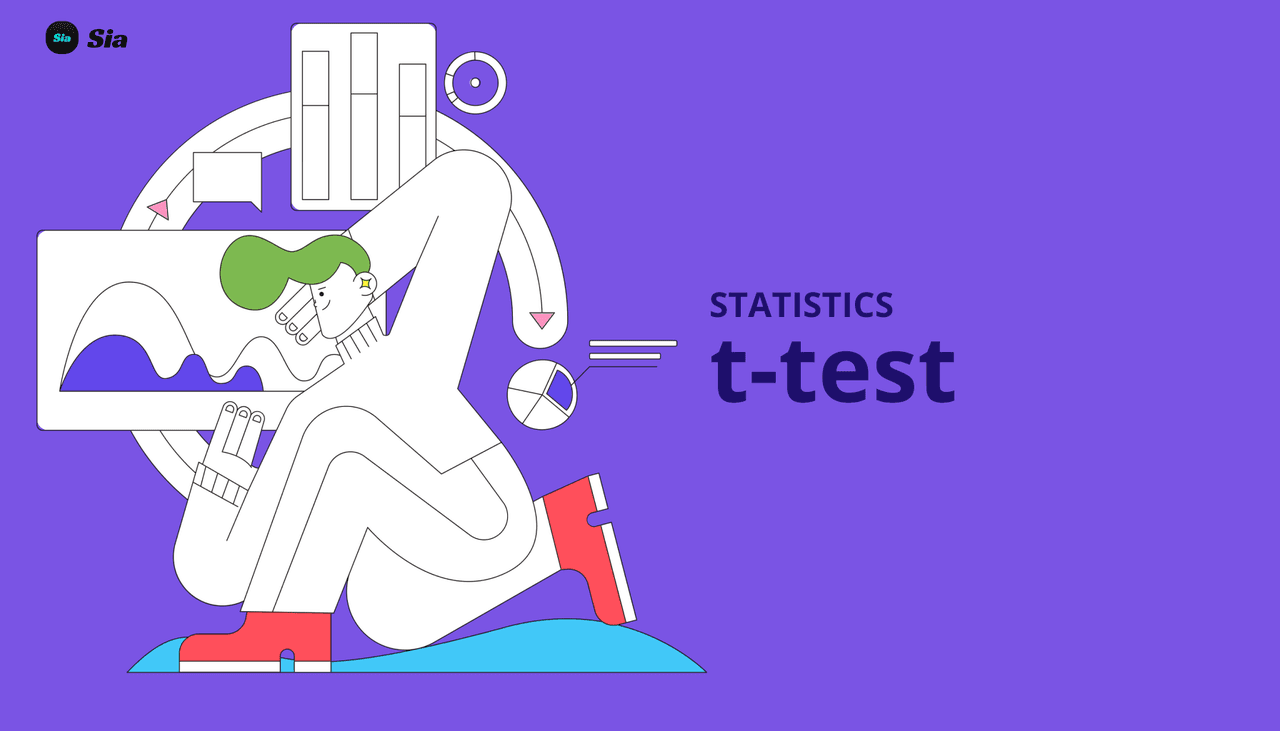
Student Question
What's t-test?
Solution by Steps
-
Step 1: Understand the purpose of a t-test: A t-test is a statistical test used to compare the means of two groups or to compare a sample mean to a known value when the population variance is unknown.
-
Step 2: Identify the type of t-test: There are different types of t-tests, including the one-sample t-test, independent two-sample t-test, and paired sample t-test. The choice depends on experimental design and data.
-
Step 3: Formulate the null hypothesis (H0) and alternative hypothesis (Ha): The null hypothesis typically states that there is no effect or no difference, while the alternative hypothesis states that there is an effect or a difference.
-
Step 4: Calculate the t-statistic using the sample data. For a one-sample t-test, the formula is:
where ˉxˉ is the sample mean, μ is the population mean, s is the sample standard deviation, and n is the sample size.
-
Step 5: Determine the degrees of freedom (df): For a one-sample t-test, df=n−1. For an independent two-sample t-test, df is calculated based on the sample sizes and variances of the two groups.
-
Step 6: Find the critical value(s) and compare with the t-statistic: Using the degrees of freedom and the desired significance level (commonly 0.05), find the critical value(s) from the t-distribution table. Compare the calculated t-statistic to the critical value(s) to determine if the null hypothesis can be rejected.
-
Step 7: Interpret the results: If the t-statistic is greater than the critical value (in absolute terms) and the p-value is less than the significance level, reject the null hypothesis. Otherwise, do not reject the null hypothesis.
Answer
The t-test is a method for testing whether there is a significant difference between means or whether a sample mean significantly differs from a known value, based on the t-distribution.
Key Concept
t-test
Explanation
A t-test is used to determine if there are significant differences between the means of two groups or between a sample mean and a known population mean, especially when the population variance is unknown. It involves calculating a t-statistic and comparing it to a critical value from the t-distribution.